Mainstreaming Technology Assisted Review
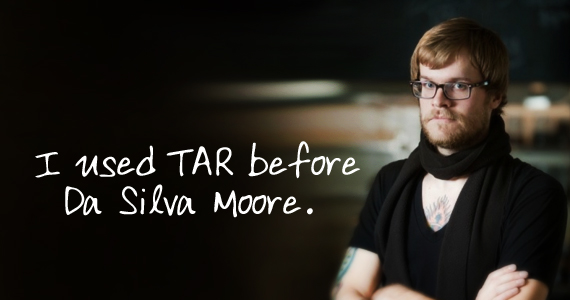
Sorry hipsters, technology-assisted review (TAR) is now mainstream.
In 2012 there have been four cases in which technology-assisted review has been in judicially endorsed in some form or another:
- Da Silva Moore v. Publicis Group (acknowledging party agreement to use TAR)
- In re Actos (Pioglitazone) Products Liability Litigation (instructing parties to meet and confer on how to implement TAR)
- Global Aerospace v. Landow Aviation (ordering the use of TAR over plaintiff’s objections)
- EORHB, Inc., et al. v. HOA Holdings, LLC (ordering the use of TAR on the court’s own initiative)
And for the not-so-early adopters? While there’s much catching up to do, here are a few choice tips that will help you build your baseline knowledge.
The Vernacular of Technology-Assisted Review
The ediscovery community loves this technology… it just doesn’t know what to call it. Here’s a smattering of the many terms floating around: “technology assisted review” (TAR), “computer assisted review” (CAR), “automated document review,” and “predictive priority.” If you’re feeling particularly vocal about your preferred jargon, if your TAR is sticky puns are just too good to lose, or if you just like clicking things, be sure to make your voice heard on the TAR v. CAR acronym poll hosted by our friends at the e-discovery team. We will get to a standard term eventually--for now, the important things are: 1) the technology behind the lingo and 2) that you, your firm, your adversary, and the court actually know what you mean when you say, for example, “computer assisted review.”
Machine Learning
I know—it’s a little scary, but machine learning is a key component of TAR. Machine learning uses advanced predictive algorithms and analytics to supplement the judgment of expert human reviewers regarding the responsiveness and non-responsiveness (or other defined category) of documents. Why won’t your TAR case fall victim to the HAL 9000 of document review you ask? Because your brightest subject matter experts will train the machine and iterative quality control checks will ensure that everything is going as planned. Bottom line: it’s a delicate dance—let the computers do what they do best and let the humans do what they do best.
Predictive Algorithms
If you’ve dabbled in consumer technology in the past 10 years, I have good news for you: the predictive component of TAR is not new. In fact, Netflix, amazon.com and online advertisements (to name a few) all employ algorithms that use your input (e.g., movies watched or products purchased) to recommend future movies or products that you might enjoy. TAR uses this same basic premise.
Key Terminology
Testing and Quality Control procedures are critical when implementing TAR. Using the following metrics, we can “score” the effectiveness of the TAR results to accept a project as complete, or to know when to keep TARing. A good key word search process will use the same methodology.
- Recall – a measure of completeness (actually relevant documents retrieved/total actually relevant documents)
- Precision – a measure of exactness or how “dirty” your search is (actually relevant documents retrieved/ total retrieved documents).
Here’s a no-frills example (assume the black box represents the documents your search identifies as responsive):